AI Risk Management Playbook
A comprehensive guide for organizations implementing AI systems, with actionable frameworks and best practices.
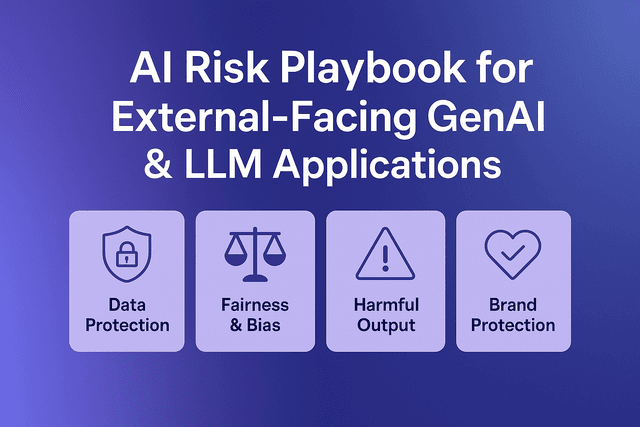
AI Risk Management Playbook
v1.0 • 37 pages
Table of Contents
1. Introduction
External-facing Generative AI (GenAI) and Large Language Model (LLM) applications introduce unique risks and regulatory obligations. This playbook provides a comprehensive AI Risk & Compliance framework tailored to such applications, drawing on international standards and laws (ISO/IEC 42001, NIST AI RMF, GDPR, EU AI Act, etc.).
Download the playbook to access all content
2. Core AI Risk and Compliance Principles
Before diving into lifecycle phases, it's crucial to establish the control objectives that anchor a robust AI risk program. These objectives – derived from frameworks for trustworthy AI – ensure your GenAI application is ethical, safe, and compliant by design.
Download the playbook to access all content
3. Planning & Design Phase
In the planning stage, the focus is on setting requirements and governance structures before any code or model is built. Early planning ensures that risk considerations drive the project from the start. This phase aligns with NIST's Govern and Map functions – establishing accountability and identifying risks upfront.
Download the playbook to access all content
4. Development (Build) Phase
During development, the team collects and prepares data, builds or fine-tunes the model, and writes the application code integrating the model. This phase is about implementing controls in the data and model. It corresponds to executing the plan ("Do" in ISO's PDCA, and partly NIST's Map and Measure functions as you quantify risks during model building). The goal is to embed ethical and compliance considerations into the model from the start, not after the fact.
Download the playbook to access all content
5. Testing & Validation Phase
Before deploying the AI system, it must undergo comprehensive testing not just for functionality, but for risk-related criteria: fairness, privacy, security, explainability, and overall regulatory compliance. This phase corresponds well with NIST's Measure function, where you evaluate and quantify risks and performance. It's also part of the "Check" in ISO's PDCA cycle – checking that the system as built meets the planned requirements. Testing should involve cross-functional reviewers (to cover technical and compliance perspectives) and realistically simulate the production environment.
Download the playbook to access all content
6. Deployment Phase
Deployment is when the AI system is released into the real world – whether that's a production environment for end-users or an internal rollout. This phase is critical: it's where all controls meet reality. The focus here is on safe launch, transparency to users, and establishing monitoring. It aligns with NIST's Manage function (putting risk mitigations into action and preparing for ongoing oversight). Even after thorough testing, deployment should be done thoughtfully, often incrementally, to ensure that any unforeseen issues can be caught early.
Download the playbook to access all content
7. Monitoring & Evolution Phase
Once deployed, the AI system enters the monitoring stage, which is effectively ongoing until the system is decommissioned. This phase is critical for sustaining compliance and performance. Models can drift, new risks can emerge, and regulations can change – so a continuous oversight process is needed. This corresponds to the remaining part of NIST's Manage function (continuous monitoring and improvement) and the "Act" in the PDCA cycle of ISO 42001 (taking corrective actions and improving the system). In practical terms, this phase involves tracking metrics, responding to incidents, refining the model or controls, and ensuring the AI continues to meet its objectives over time.
Download the playbook to access all content
8. Conclusion and Next Steps
Developing and deploying external-facing GenAI and LLM applications comes with significant responsibilities – but by following this playbook, organizations can confidently navigate the intersection of innovation and compliance. We covered the entire AI lifecycle from planning to monitoring, mapped against leading frameworks (ISO 42001, NIST AI RMF, GDPR, EU AI Act, etc.), and provided actionable checklists and control examples. Strategic alignment with these frameworks ensures that your AI initiatives are built on a foundation of trustworthiness and accountability. Meanwhile, the practical tools – control objectives, lifecycle checklists, embedded control-as-code controls, and metrics – make those high-level principles concrete and measurable in day-to-day AI development operations.
Download the playbook to access all content
Implement AI Risk Management with Tavo
Take the next step in your AI governance journey. Tavo's platform helps you put the principles from this playbook into practice with automated risk assessment, policy management, and continuous monitoring.